Yixuan (Cassie) Song is a PhD candidate in materials science and engineering who is investigating novel phenomena enabled by special magnetic materials, termed ferrimagnets, in which the magnetism of neighboring atoms differs in strength and points in opposite directions. Supported by a MathWorks Fellowship, Cassie will pursue research to better understand this unique property, which promises a much more effective method for developing devices that perform data storage and other spintronics phenomena. Cassie has developed an algorithm for a macrospin model to simulate the magnetization reversal process in systems with complex anisotropy landscapes. Other important areas of inquiry in her research are the magnetic dynamics in the frequency range of GHz to THz, which may have important implications for future technologies, and demagnetization fields in magnetic materials that exhibit asymmetric shapes, such as thin films and cylinders. Using MATLAB to conduct the finite element analysis plays a central role in Cassie’s work. Her research has the potential to deliver important new knowledge and design approaches to advance the field of spintronics and terahertz materials science.
https://engineering.mit.edu/wp-content/uploads/2024/10/headshot_Song_Yixuan_Cassie_Mathworks_SoE_2025.jpg
Website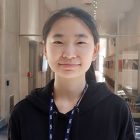
Yixuan (Cassie) Song
Materials Science and Engineering https://engineering.mit.edu/fellows/yixuan-cassie-song/Mayuri Sridhar is a PhD candidate in electrical engineering and computer science who focuses on privacy-preserving computation. As a MathWorks Fellow, Mayuri will expand her work on providing statistical guarantees regarding robustness and security for algorithms in realistic deployed settings. Her recent work focuses on PAC privacy, where she has shown the intrinsic relationship between the stability of algorithms and their potential for privatization. This work provides a tight theoretical characterization of the noise required to privatize any algorithm as a function of its covariance, along with extensive experimental results validating the utility of these algorithms. In particular, she shows that privatizing complex algorithms like SVM or K-Means can be done in a black-box manner with a negligible impact on efficacy. MATLAB’s Parallel Computing Toolbox and Deep Learning Toolbox could enable her to extend her work to more complex models like BERT or even GPT. Mayuri’s work has strong potential to advance privacy-preserving computing with broad applications, from providing privacy guarantees when interacting with large public models like ChatGPT to robust learning guarantees on sensitive medical and financial data.
https://engineering.mit.edu/wp-content/uploads/2024/10/headshot_Sridhar_Mayuri_Mathworks_EECS_2025.jpg
Website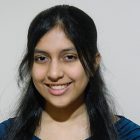
Mayuri Sridhar
Electrical Engineering and Computer Science https://engineering.mit.edu/fellows/mayuri-sridhar/Stephan Stansfield is a PhD candidate in mechanical engineering who conducts basic research to understand the neural control of movement and applied research to develop assistive devices. His MathWorks Fellowship will support work in two areas. The first is a study of human interactions with dynamically complex objects, combining human subject experiments with MATLAB-based simulations using an input-shaping control strategy. His results suggest that that the brain may greatly simplify representations of flexible object dynamics during manipulation and exploit mechanical impedance to deal with errors. Stephan’s second project involves the development of an assistive device for older adults to help them stand up and sit down with the goal of preserving independence and preventing falls. He has created a soft, wearable “exosuit” and is preparing for human subject studies to characterize device interactions with the body. During these experiments, he will gather motion capture, force plate, and EMG data to analyze and visualize with MATLAB. Stephan’s work has significant promise to deepen our understanding of neuromotor control and advance new assistive robotic devices that could improve health and quality of life.
https://engineering.mit.edu/wp-content/uploads/2024/10/headshot_Stansfield_Stephan_Mathworks_MechE_2025.jpg
Website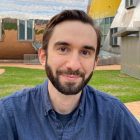
Stephan Stansfield
Mechanical Engineering https://engineering.mit.edu/fellows/stephan-stansfield/Soumya Sudhakar is a PhD candidate in aeronautics and astronautics whose research is focused on decision-making algorithms for miniature or long-duration robots that are energy- efficient in actuation and computing. As a MathWorks Fellow, she will investigate new methods for measuring uncertainty in machine learning in a computationally efficient way. To address the high computational costs of current methods, Soumya has proposed a novel method to exploit temporal correlation across the inputs such that only a single inference is required per input, for much greater computational efficiency. She is also investigating efficient online learning, an essential problem for robots with limited resources in the era of extremely large AI models, as well as the topic of computing’s climate impact, an important subject given the large amount of computing required for the navigation of autonomous vehicles. Soumya’s research makes significant use of MATLAB, and her research offers exciting new directions in energy-constrained perception, planning, and actuation to advance miniature and long-duration robotics in novel applications.
https://engineering.mit.edu/wp-content/uploads/2024/10/headshot_Sudhakar_Soumya_Mathworks_AeroAstro_2025.jpg
Website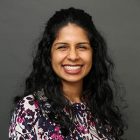
Soumya Sudhakar
Aeronautics and Astronautics https://engineering.mit.edu/fellows/soumya-sudhakar/Anantha Narayanan Suresh Babu is a PhD candidate in mechanical engineering whose research is focused on stochastic modeling and Bayesian learning of sea ice dynamics, as well as scientific deep learning and neural closure modeling for ocean dynamics. Anantha’s second MathWorks Fellowship will enable him to extend this work. His first focus will be utilizing a MATLAB-based Gaussian mixture model filtering and model learning framework for discriminating between competing sea ice model formulations and discovering new models from data. His second focus will be developing and refining physics-inspired neural architectures and training methodologies to model complex fluid and oceanic processes, including sea ice. By combining the strengths of physics-based numerical modeling with the adaptability and efficiency of deep learning, the resulting neural closure models promise enhanced accuracy and predictive capabilities at a significantly reduced computational cost. Anantha’s work holds the potential to advance our understanding of sea ice and ocean dynamics, which could have applications in climate modeling, environmental monitoring and forecasting, marine ecosystem protection, resource management, maritime transport, and policy and decision-making for the future of our planet.
https://engineering.mit.edu/wp-content/uploads/2024/10/headshot_Anantha_Narayanan_Suresh_Babu_MechE_2025.jpg
Website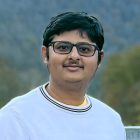
Anantha Narayanan Suresh Babu
Mechanical Engineering https://engineering.mit.edu/fellows/anantha-narayanan-suresh-babu-2/Nishat Tabassum is a PhD candidate in chemical engineering whose research aims to assess the feasibility of engineering small proteins as alternatives to monoclonal antibody therapeutics. Specifically, Nishat is working to design a non-immunoglobulin protein that targets the cytokine tumor necrosis factor-alpha and prevents it from binding to its receptors, thus preventing an inflammatory response. A common challenge in treating chronic inflammation in autoimmune diseases is that patients may become unresponsive to immunoglobulin-based treatments. Non- immunoglobulin proteins are promising alternatives as they are small and easily produced in peptide synthesizers. Nishat has developed a preliminary model that can significantly speed the identification of promising design targets and offers a clearer understanding of which parameters must be optimized for binder design. As a MathWorks Fellow, Nishat will further develop this model into a more detailed physiologically based pharmacokinetic model with results obtained experimentally. Her work has the potential to advance powerful new therapies for autoimmune diseases, and the MATLAB models and methods she has pioneered may aid other researchers in informing design targets for protein engineering in drug development.
https://engineering.mit.edu/wp-content/uploads/2024/10/headshot_Tabassum_Nishat_Mathworks_ChemE_2025.jpg
Website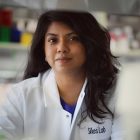
Nishat Tabassum
Chemical Engineering https://engineering.mit.edu/fellows/nishat-tabassum/Hao Tang is a PhD candidate in materials science and engineering whose research is focused on developing and applying computational methods to simulate physical systems ranging from quantum defects and functional alloys to electronic devices. A second MathWorks Fellowship will enable Hao to extend his work, which draws significantly on MathWorks toolkits for modeling, simulations, data analysis, and visualization. His recent and current projects include: the refinement of a new reinforcement learning method to accelerate the atomistic simulation of defect diffusion; the application of an equivariant graph neural network to improve the accuracy of electronic structure calculations of molecules; the development of a computational method to calculate color centers’ quantum sensing performance; and the creation of new computational methods for evaluating the energy levels, wavefunctions, and lifetime of neutron bound states. Hao’s research has already delivered new discoveries and methods in computational materials science, and his ongoing work has strong potential to push the boundaries of his field while also offering computational tools of value to researchers in many disciplines.
https://engineering.mit.edu/wp-content/uploads/2024/10/headshot_Tang_Hao_Mathworks_SoE_2025.jpg
Website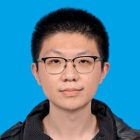
Hao Tang
Materials Science and Engineering https://engineering.mit.edu/fellows/hao-tang-2/Jacob Toney is a PhD candidate in chemical engineering whose research aims to develop machine learning-accelerated quantum chemistry tools. As a MathWorks Fellow, Jacob will pursue several objectives, the first of which is building deep-learning models to predict these catalyst structures and their reactive intermediates. He has already created a model capable of predicting ligand denticity and coordinating atoms with very high accuracy. Jacob is building tools to characterize the transition states of these catalysts, once formed, to predict rates of catalysis and deduce mechanisms. Next, Jacob will use the deduced structures and mechanisms to predict and optimize catalysts based on datasets of experimental outcomes. Finally, Jacob is leading projects aimed at unlocking greater predictive power from deep-learning models trained to predict the properties of transition metal complexes. Jacob’s research offers key insights into how catalyst structures inform reaction outcomes and is producing tools broadly useful to those working on catalyst design. His work also has potentially significant impacts on industry and the enhancement of materials syntheses using organometallic chemistry and could serve to make these processes more productive and energy efficient.
https://engineering.mit.edu/wp-content/uploads/2024/10/headshot_Toney_Jacob_Mathworks_ChemE_2025.jpg
Website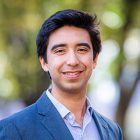
Jacob Toney
Chemical Engineering https://engineering.mit.edu/fellows/jacob-toney/Julian Ufert is a PhD student in chemical engineering whose research is focused on ethylene production, the second-highest greenhouse gas emitter in the chemical industry and ubiquitous in manufacturing around the globe. As a MathWorks Fellow, Julian aims to develop a clean, economical ethylene production process utilizing novel high- and intermediate-temperature electrochemical approaches, which may offer a way to electrify the process while also coping with byproduct and separation issues. Primary objectives of his project include conducting techno- economic analysis and life-cycle assessments of the synergistic integration of steam crackers with existing and technologically mature processes and developing two novel catalytic routes to ethylene production—electrochemical oxidative coupling of methane and electrochemically assisted ethane dehydrogenation—that are selective and inherently electrified. Julian draws on MATLAB for many aspects of this research and is creating reactor models and other MathWorks tools of prospective value for other researchers. By designing new, clean ethylene production methods that are economically competitive with incumbent technology, Julian’s research work has significant potential to help decarbonize the chemical industry.
https://engineering.mit.edu/wp-content/uploads/2024/10/headshot_Ufert_Julian_Mathworks_ChemE_2025.jpg
Website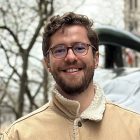
Julian Ufert
Chemical Engineering https://engineering.mit.edu/fellows/julian-ufert/Ilan M. L. Upfal is a PhD student in civil and environmental engineering whose research focuses on sustainable energy system design. With the support of a MathWorks Fellowship, Ilan will expand his work in wind farm design and translate those efforts into industry-ready MATLAB tools. His research aims to consider collective flow control in the optimization of wind farm layouts. Ilan’s work could include two valuable outcomes: a gradient-based optimization approach utilizing automatic differentiation to efficiently solve the high-dimensional control and layout optimization problem, which otherwise would be computationally infeasible using finite-difference-based optimizers, and a physics-based modeling approach that uses a generalized momentum model to accurately predict the impact of turbine control on wind farm power production. Ilan has demonstrated that considering collective flow control during wind farm design yields significantly smaller land-area footprints while reducing the cost of wind energy and increasing the lifetime profit of wind farms. Ilan’s research has the potential to advance wind power that maximally accelerates decarbonization, improves grid reliability, and provides valuable tools for research in aerodynamics, modeling, control, and sustainable power.
https://engineering.mit.edu/wp-content/uploads/2024/10/headshot_Upfal_Ilan_Mathworks_CEE_2025.jpg
Website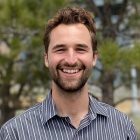
Ilan M. L. Upfal
Civil and Environmental Engineering https://engineering.mit.edu/fellows/ilan-m-l-upfal/Thomas W. O. Varnish is a PhD candidate in nuclear science and engineering whose research focuses on magnetic reconnection, an explosive reconfiguration of magnetic field topology in a plasma that rapidly dissipates magnetic energy by heating and accelerating the plasma. Specifically, Thomas is exploring a special case of this process known as guide-field magnetic reconnection, in which a component of the magnetic field is directed out of the plane of reconnection. As a MathWorks Fellow, he is playing a lead role in the construction of PUFFIN, a new microsecond pulsed-power generator that will enable the first quasi-steady-state studies of many plasma phenomena. Through PUFFIN, Thomas will study how the stability and evolution of the reconnection layer are affected by a guide field and will implement advanced diagnostics such as Faraday imaging. MATLAB Simulink and Simuscape are essential to PUFFIN’s construction and Thomas’s work in general. His research has the potential to offer important insights into magnetic reconnection and plasma physics and associated phenomena such as solar flares, black hole accretion disks, and supernovae.
https://engineering.mit.edu/wp-content/uploads/2024/10/headshot_Varnish_Thomas_Mathworks_NSE_2025.jpg
Website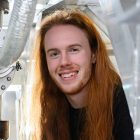
Thomas W. O. Varnish
Nuclear Science and Engineering https://engineering.mit.edu/fellows/thomas-w-o-varnish/Alexander A. Velberg is a PhD student in nuclear science and engineering whose research lies at the interface of machine learning and nonlinear plasma dynamics. Specifically, Alex aims to develop a systematic program of data-driven equation discovery for plasma physics. His work builds on recent research demonstrating that it is possible to discover a known reduced plasma model from first-principles simulation data. Alex is exploring how to extend this methodology to the discovery of equations where some terms are unknown, namely, the data-driven discovery of so-called sub-grid closures. MATLAB’s Deep Learning Toolbox is the main research platform for this project. First-principles descriptions, including the detailed physical effects of discrete particles in plasmas, are extremely computationally intensive; therefore, the ability to accurately represent those effects in terms of an effective closure with a significantly reduced computational cost, would be transformational in terms of our ability to simulate plasmas. Alex’s work has the potential to offer these powerful capabilities and help usher in a new era of exploration in plasma physics, with applications to astrophysical plasmas and fusion energy.
https://engineering.mit.edu/wp-content/uploads/2024/10/headshot_Velberg_Alexander_Mathworks_NSE_2025.jpg
Website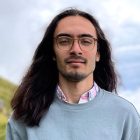
Alexander A. Velberg
Nuclear Science and Engineering https://engineering.mit.edu/fellows/alexander-a-velberg/Trent Weiss is a PhD candidate in chemical engineering whose research is focused on electrochemical technologies, particularly lithium-ion batteries (LIBs), a promising technology for decarbonizing transportation, energy, and other sectors. As a MathWorks Fellow, Trent will address shortfalls in current LIBs using MATLAB-based modeling to explore an alternate cell-level architecture with the aim of developing a convection-enhanced LIB with improved storage capacity and charging/discharge rates. A central aim of his project is to advance our understanding of how convection improves mass and thermal transport. His future objectives include explorations of the structure-property relations for convection battery electrodes and identifying trends that inform specific values for different chemistries, cell configurations, and applications to reduce the experimental design space. His work in conventional LIBs may be generalizable to other intercalation chemistries, such as alternative metal-ion batteries and redox reservoirs useful for electrochemical energy storage or ion separation. Trent’s research has the potential to advance new concepts for electrochemical energy storage, thereby helping to enable the deep decarbonization of electricity generation, transportation, and industrial processes.
https://engineering.mit.edu/wp-content/uploads/2024/10/headshot_Weiss_Trent_Mathworks_ChemE_2025.jpg
Website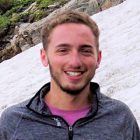
Trent Weiss
Chemical Engineering https://engineering.mit.edu/fellows/trent-weiss/Duo Xu is a PhD candidate in mechanical engineering whose research integrates materials science, heat transfer, and radiation transport in the development of polymer-based materials. His prior research included the creation of a polyethylene-based multifunctional composite material for space exploration; testing and modeling of an olefin block copolymer material for solid-state thermal management by mechanocaloric effect; and demonstrating strain-induced tunable thermal conductivity in the same olefin block copolymer, supported by a proposed theoretical explanation. MATLAB-based computational tools developed by Duo were pivotal in preparing materials from his lab. The multifunctional composite material he developed was sent for testing under real conditions on a recent Axiom Ax-2 mission launch. His second MathWorks Fellowship will support his work to create new elastic fibers for active and passive thermoregulation technologies and to explore methods like radiation crosslinking to enhance the reversible mechanical properties of materials he developed without compromising their performance and recyclability. Duo’s current and future research has excellent potential to advance materials that will have a global impact on energy efficiency and sustainability.
https://engineering.mit.edu/wp-content/uploads/2024/10/headshot_Xu_Duo_Mathworks_MechE_2025.jpg
Website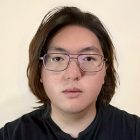
Duo Xu
Mechanical Engineering https://engineering.mit.edu/fellows/duo-xu-2/Haike Xu is a PhD candidate in electrical engineering and computer science whose research is focused on analyzing and designing algorithms. With the vast amount of data generated and collected today, nearest-neighbor search algorithms find applications in various domains, from constructing information retrieval systems to enhancing large language models for improved factuality. Supported by a MathWorks Fellowship, Haike will expand on his successful work on the nearest neighbor search problem, including building a theoretical understanding of why one such algorithm, DiskANN, is more efficient in practice than in theory, and identifying the relevant properties of datasets for which the algorithm is efficient. Going forward, Haike plans to explore additional new directions in nearest neighbor search, such as efficiently searching according to non-similarity-based metrics and constructing search indices with limited space and time resources. His research holds the potential to offer valuable new directions in algorithm analysis and design and serve broader needs for fast, efficient data processing and computing for research and industry.
https://engineering.mit.edu/wp-content/uploads/2024/10/headshot_Xu_Haike_Mathworks_EECS_2025.jpg
Website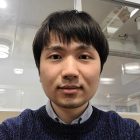
Haike Xu
Electrical Engineering and Computer Science https://engineering.mit.edu/fellows/haike-xu/Zi Yu (Fisher) Xue is a PhD candidate in electrical engineering and computer science whose research addresses the critical need for energy-efficient and high-performance computing systems. Specifically, Fisher’s work focuses on applications of tensor algebra, a computing paradigm used across a wide variety of application domains, including scientific simulations, data analytics, and recommendation systems. As a MathWorks Fellow, Fisher will focus on designing new architectures to better perform sparse computation for both existing and emerging applications, with the goal of developing more advanced hardware that enables more efficient computational systems. Recently, he has demonstrated the viability of overbooking-based speculation in sparse tensor algebra accelerators, an innovative approach that could support more efficient hardware design. His current work focuses on designing hardware to enable larger and more accurate symmetry-equivariant neural networks, which efficiently represent interactions of physical systems and thus have both extreme data efficiency and robust generalization when modeling systems such as molecules, airfoils, climates, or galaxies. Fisher’s work has strong potential to support the development of essential hardware for computing systems combining high performance and energy efficiency.
https://engineering.mit.edu/wp-content/uploads/2024/10/headshot_Xue_Zi_Yu_Fisher_Mathworks_SoE_2025.jpg
Website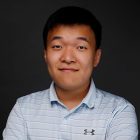
Zi Yu (Fisher) Xue
Electrical Engineering and Computer Science https://engineering.mit.edu/fellows/zi-yu-fisher-xue/Sungyun Yang is a PhD candidate in chemical engineering whose multidisciplinary research aims to develop innovative new approaches in pharmacokinetic modeling, materials science, and micro-robotics for biomedical applications. As a MathWorks Fellow, Sungyun will expand his work in several areas, including MATLAB-based simulations of physiological glucoregulatory systems to develop novel insulin therapeutics and designing colloidal-scale microrobots and microscopic sensors for deployment in confined fluidic environments such as microfluidics or circulatory systems. His current objectives include expanding the glucoregulatory system model from human models to other animal model species and enhancing the model with extended hepatic functions to capture complex dynamics, such as the mixed signaling of glucagon and insulin, and glycogen dynamics. Sungyun’s work is yielding novel insights and applications in multiple areas, from the development of novel 2D materials to new directions in diabetes treatment to highly original design strategies in colloidal-scale microrobots. In addition, the methods and tools Sungyun is creating to support his work hold great promise to advance research more broadly in chemical engineering, materials science, and biological engineering.
https://engineering.mit.edu/wp-content/uploads/2024/10/headshot_Yang_Sungyun_Mathworks_ChemE_2025.jpg
Website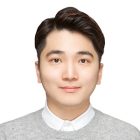
Sungyun Yang
Chemical Engineering https://engineering.mit.edu/fellows/sungyun-yang/Yuheng Yang is a PhD student in electrical engineering and computer science whose research is focused on the development of end-to-end security verification frameworks for hardware designs. With the support of a MathWorks Fellowship, Yuheng will work to bridge the gap between computer architecture and formal verification, making formal tools easily accessible to hardware designers, including tools for both the early and later register-transfer level (RTL) stages. Currently, he is exploring verification techniques targeting RTL implementations. Yuheng’s vision is to innovate verification schemes, achieving better scalability by leveraging architectural insights, so that hardware designers can be involved in the verification loop. To that end, he is designing verification schemes for secure speculation defenses with two objectives: to allow hardware designers to assist the verification process by writing shadow logic machinery interacting with processor logic and to boost the verification scalability by exploring the taint tracking technique. Yuheng’s research could significantly contribute to MathWorks’s HDL tools by improving how the industry approaches hardware design through more effective methods and greater involvement of hardware engineers in the verification process.
https://engineering.mit.edu/wp-content/uploads/2024/10/headshot_Yang_Yuheng_Mathworks_EECS_2025.jpg
Website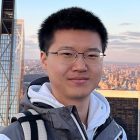
Yuheng Yang
Electrical Engineering and Computer Science https://engineering.mit.edu/fellows/yuheng-yang/Lale Yilmaz is a PhD candidate in mechanical engineering whose research focuses on nonlinear mechanics and granular media. Specifically, she is working to develop simulation tools to model uprooting processes in different soils and has created a MATLAB-based software package that combines nonlinear beam theory and a novel variant of the Resistive Force Theory (RFT) of granular media, enabling her to create the first simulations of flexible root-like structures that interact mechanically with soil during the process of uprooting. The resulting elastic RFT (eRFT) incorporates elasticity and captures the resistance provided by the granular soils in uprooting systems. As a MathWorks Fellow, Lale plans to adapt this paired eRFT-inextensible roots solver to more complex geometries, using a MATLAB-based root geometry generator she has created to manipulate the defining parameters of the root structure. She will also introduce viscosity to the solver and extend it to a wider range of soil models. Lale’s research has the potential to offer next- generation computational engineering tools supporting research in many complex systems and phenomena.
https://engineering.mit.edu/wp-content/uploads/2024/10/headshot_Yilmaz_Lale_Mathworks_MechE_2025.jpg
Website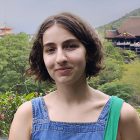
Lale Yilmaz
Mechanical Engineering https://engineering.mit.edu/fellows/lale-yilmaz/Nomi Yu is a graduate student in mechanical engineering whose research utilizes deep learning techniques to innovate the mechanical design process and to expand AI capabilities in design and manufacturing. As a MathWorks Fellow, Nomi will pursue two primary projects with the objective of creating models of additive manufacturing processes to predict the “manufacturability” of various geometries and incorporating these models in generative design tools. The first project aims to build a differentiable model for manufacturability-rule prediction, incorporating heuristic-based design rules to assess the printability of a given geometry. The second project seeks to produce an empirical model from scanned 3D prints to explore the potential of deep learning models to detect features associated with poor manufacturability that people may have a harder time noticing. Nomi makes extensive use of MATLAB and plans to make valuable contributions to the MATLAB community, including the implementation of 3D deep-learning networks and a centralized toolbox for geometric analysis. Their research could deliver novel techniques and insights with the potential to elevate design and manufacturing through AI and deep learning.
https://engineering.mit.edu/wp-content/uploads/2024/10/headshot_Yu_Nomi_Mathworks_MechE_2025.jpg
Website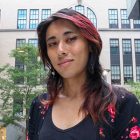