Matthew Yeung is a PhD candidate whose research explores interactions between light and nanostructures for both fundamental research and the development of novel optoelectronic technologies. The focus of his current work, supported by a MathWorks Fellowship, is the development of nanostructured devices that can interact with and measure light fields with sub-femtosecond resolution. These devices enable a critical new tool in visible to near-infrared optical metrology: a sampling oscilloscope for light waves that could offer a fresh time-domain perspective on how light interacts with materials. Matthew’s work has a large number of potential applications, including creating more efficient solar cells, understanding the fundamental energy transfer mechanisms that enable photosynthesis, and characterizing materials with unprecedented sensitivity. MATLAB has been vital in Matthew’s device design, experiment development, and instrumentation interfacing, and he looks forward to sharing the resulting tools with the MathWorks community. His work has the potential to help usher in revolutionary advances in light-based technologies.
https://engineering.mit.edu/wp-content/uploads/2023/10/Yeung_Matthew_mathworks2023_headshot.jpg
Website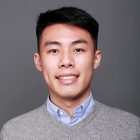
Matthew Yeung
Electrical Engineering and Computer Science https://engineering.mit.edu/fellows/matthew-yeung/Li-Yu Yu is a PhD candidate whose research seeks to advance the growing field of spectroscopic microscopy, a powerful tool for delivering rich molecular information about an object through the measurement of its spatial and spectral characteristics. Potential applications of spectral microscopy range from medical diagnosis to pharmaceutical research and material identification. Lu-Yu’s current research, supported by a MathWorks Fellowship, addresses the crucial drawback of poor spectral signal-to-noise ratio in conventional dispersive spectrometers and limited spectral tuning capability in conventional laser sources. His goal is to develop a new spectroscopic measurement strategy that leverages illumination encoding and model-based AI to achieve broadband, real-time, super-resolved spatiospectral image reconstruction, offering a platform for advanced functional analysis in a sophisticated multicellular environment. MATLAB has been an essential tool in this work, enabling the simulation of optical physics, optimization, signal processing, and machine learning. Li-Yu’s work has the potential for far-reaching impact in the field of spectroscopic microscopy and could deliver useful new perspectives and open-source data processing tools (including tools to support a core data processing challenge of compressive sensing and spectral unmixing) to advance innovative research across the imaging and sensing, and signal processing communities.
https://engineering.mit.edu/wp-content/uploads/2023/10/Yu_Li-Yu_mathworks2023_headshot.jpg
Website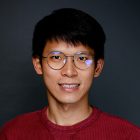
Li-Yu Yu
Electrical Engineering and Computer Science https://engineering.mit.edu/fellows/li-yu-yu/Mingxin Yu is a PhD candidate whose research is in the fields of robotics and machine learning. As a MathWorks Fellow, Mingxin will contribute to a research program aimed at developing a learning-based method for accelerating motion planning in robot manipulation problems and will help to develop a proposed, new approach that leverages a machine learning-based control barrier functions (CBF) in safety-critical motion planning scenarios to tackle the high sampling complexity of traditional sampling-based motion planning methods. The principal aim of this project is to incorporate CBF into the traditional motion planning methods to steer the system to navigate safely to the goal, while significantly reducing time-consuming sampling tasks and maintaining the guarantees of the original methods. Mingxin’s research has the potential to offer valuable new solutions to a planning problem with significant impact on numerous real-world applications, including manipulation in cluttered environments, multi-arm assembly tasks, and human-robot interaction.
https://engineering.mit.edu/wp-content/uploads/2023/10/Yu_Mingxin_mathworks2023_headshot.jpg
Website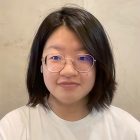
Mingxin Yu
Aeronautics and Astronautics https://engineering.mit.edu/fellows/mingxin-yu/James H. Zhang is a PhD candidate whose research concentrates on efficient, economical strategies for desalinating water using porous, interfacial evaporators. This technology utilizes solar energy to evaporate water from brackish sources wicked inside of a interfacial evaporator. The vapor can then be collected and condensed into clean water. Specifically, James is exploring the thermodynamics of solar-driven evaporation inside porous materials, particularly in the case of super-thermal evaporation rates (2-3 times beyond the thermal limit), to optimize porous materials for use in desalination devices. He has designed a MATLAB-based model to investigate the evaporation process and identify key variables that could be manipulated to maximize evaporation rates. The model, combined with a MATLAB model of light-structure interactions developed by James’s mentee, has the potential to yield a comprehensive understanding of transport phenomena in porous evaporators. A MathWorks Fellowship will enable James to continue his research in super-thermal evaporation rates, which will be critical in the development of desalination technology and could help the growing global need for efficient clean water production.
https://engineering.mit.edu/wp-content/uploads/2023/10/Zhang_James-H_mathworks2023_headshot.jpg
Website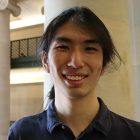
James H. Zhang
Mechanical Engineering https://engineering.mit.edu/fellows/james-h-zhang/Ruiqi Zhang is a PhD candidate whose research aims to develop novel solar cell technologies to support renewable energy. Specifically, he is devising machine learning (ML) algorithms to predict optimal structures for solar photovoltaic devices that utilize organic-metal-halide perovskites, a class of materials with promising optoelectronic properties for the next generation of solar photovoltaics. Supported by a MathWorks Fellowship, Ruiqi will pursue a research project with the aim of connecting the physical properties of perovskite materials to their operation in completed solar structures. MATLAB is an essential tool in all four phases of this project: photovoltaic device fabrication, data acquisition and processing, physical model development, and ML algorithm development. His predictive work has demonstrated less than 5% between predicted outputs and ground truth performance—a remarkable indicator of potential success. Ruiqi’s work promises to enable rapid, targeted investigations of various novel perovskite compositions and identify the optimal compositions for perovskite solar structures. Ultimately, his work could represent a major step forward in solar energy, helping to meet the need for clean, renewable power.
https://engineering.mit.edu/wp-content/uploads/2023/10/Zhang_Ruiqi_mathworks2023_headshot.jpg
Website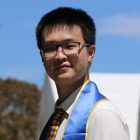
Ruiqi Zhang
Electrical Engineering and Computer Science https://engineering.mit.edu/fellows/ruiqi-zhang/Tian Zhao is a PhD candidate in the field of environmental fluid mechanics whose research focuses on fluid motion and sediment transport in aquatic ecosystems, which has critical implications for natural habitat provision, water quality protection, flood and erosion mitigation, and carbon sequestration. Supported by a MathWorks Fellowship, Tian is investigating the fundamental hydrodynamic processes in this complex system to understand how aquatic vegetation, like seagrasses or mangroves, interact with flow and sediment and, in turn, how these outcomes affect sediment transport, erosion, and deposition. MathWorks tools enable Tian to work with complex velocity datasets and simulate flow through aquatic canopies with varying geometries. By expanding our understanding of these important earth surface processes, Tian’s work could advance the restoration and building of green infrastructures in coastal and alluvial systems and improve the prediction of restoration outcomes, potentially impacting the lives and livelihoods of millions around the globe.
https://engineering.mit.edu/wp-content/uploads/2023/10/Zhao_Tian_mathworks2023_headshot.jpg
Website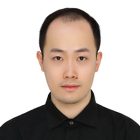
Tian Zhao
Civil and Environmental Engineering https://engineering.mit.edu/fellows/tian-zhao/Xingcheng Zhou is a PhD candidate whose research focuses on developing simple, accurate, and economical electrochemical diagnostics, which are especially crucial for healthcare in low-resource settings. Supported by her second MathWorks Fellowship, Xingcheng will extend her productive work on electrochemical sensors to diagnose bacterial and viral infectious diseases by conjugating biomolecules on the surface of electrodes to capture disease biomarkers and convert the capture to an electrical signal. Additionally, she aims to use statistical machine learning models to determine if combinations of signal changes through SWV, CV, chronoamperometry, and electrochemical impedance spectroscopy can decrease false negative rates. MathWorks tools are foundational to Xingcheng’s research, and her shared models could be beneficial to fellow researchers in the electrochemistry community. By providing affordable, accessible, and accurate diagnostic tools, Xingcheng’s work may contribute to improved health and health equity in low-resource settings around the world.
https://engineering.mit.edu/wp-content/uploads/2023/10/Zhou_Xingcheng_CIndy_mathworks2023_headshot.jpg
Website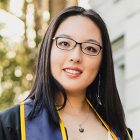